Artificial intelligence is no longer the prerogative of sci-fi movies or robot fighting games, but can help health managers predict demands and outcomes for services and distil wordy reports into two-page summaries, writes Ian Darbey.
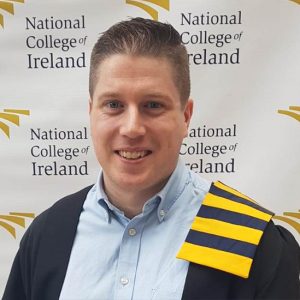
You have probably read a little about machine learning and artificial intelligence without any specific information on how they can relate to management in the healthcare sector.
For health managers the potential is quite wide ranging. It’s been shown to accurately forecast outpatient referral demand within each specialty in a large acute hospital, to enable planning. It’s been used to predict appointment DNAs and has recently been deployed in University College Hospital in London to predict patients most likely to not attend for MRI appointments who have then been targeted with reminders to reduce DNA rates and enhance key resource utilisation. Additionally, it can be used to identify influential factors, such as what makes a patient more or less likely to attend their appointment.
We were able to correctly predict over 90% of the patients who did not attend their appointments.
The terms machine learning and artificial intelligence are often inter-changeable in their everyday usage and you shouldn’t get too hung up on whether something is strictly classified as one or the other. They are grounded in statistics and at times some fairly complex mathematics. However; they are designed to improve understanding of a specific area of interest.
Cambridge Analytica is perhaps the most famous usage of machine learning and artificial intelligence, where users’ data was used to profile and distribute targeted advertising about upcoming elections to users. However, its potential usage extends far beyond advertising.
Forecasting Demand
Given the pressures on service delivery in the healthcare network, understanding demand is important. I recently completed a project which produces a 12 month forecast of outpatient demand. These forecasts are based on ARIMA modelling (often used to forecast stock prices over time).
With this, current capacity can be assessed against the backdrop of expected future demand and inform decision making around capacity and service delivery planning removing the need for any best guess on demand trends. This forecast can be set to roll over each month and provide an ongoing understanding of where demand is headed.
To date the forecasts have fallen within the forecasted range and as time goes on I’m hoping to look at other areas such as procedure waiting list growth trends, investigation ordering trends and other areas where waiting lists have developed over time.
All of the methods described above can be done within the realm of free and open source software.
Predicting Outcomes
The term “outcome” here is quite a bit broader than it would typically be in a healthcare setting. In this context, it is about classification or value prediction; will a patient be admitted or not based on their triage notes? How many patients are expected to be discharged today? Will the patient I’ve scheduled for an appointment show-up?
The final example was actual a prediction project I completed recently. Using a sample patient dataset which included age, some co-morbidities, whether the patient got an SMS reminder and the lead time between appointment booking and the appointment date we were able to correctly predict over 90% of the patients who did not attend their appointments. A similar project, with similar success rate for non attending patients, has just gone live with University College Hospital in London where the patients identified as most likely to not attend are targeted with calls and reminder texts to attend their MRI scan.
Influential Factors
As part of the development of the predictive and forecasting models often the factors which contribute most to the outcome can be identified. For example, a patient cohort with a dedicated pathway could be shown to have less variation in their length of stay vs patients without one. This can lead to change and improvement initiatives to attempt to address these issues and improve quality of care and cost effectiveness. In time related data (such as referrals and waiting list growth) the factors identified can be seasonal demand trends, which if known could be planned for.
What’s the cost?
Capital outlay can often be quite low especially for organisations who regularly replace IT infrastructure. All of the methods described above can be done within the realm of free and open source software (the R and Python programming languages are the most popular).
What’s the catch?
Skills – Finding a data analyst, data scientist or business intelligence analyst is one thing, knowing they have the healthcare domain knowledge to use their skills for you and your organisation in a way that provides the maximum impact is quite another. In the advertising, sales and financial sectors the skills and experience required are often similar or at least a closer match than when compared to someone coming into the health sector.
Data – Your analysis, predictions and forecasts will only be reliable if the data which is used to generate them is reliable and accurate. This has become less of an issue in the health sector with national reporting requirements but is obviously still an issue where information is manually recorded.
What can realistically be done?
Predictive modelling and forecasting is not out of reach. With the appropriate skills and accurate data there is a wealth of insights to be mined from the datasets within the health sector and that is even before you consider future moves towards electronic patient records which will expand the opportunities considerably.
If you often receive reports which amount to a spreadsheet containing thousands of line items you are right to wonder what benefit you are gaining from the data. A structured data analytics approach provides the opportunity to distil reports to one or two page summaries, highlighting key relationships within the data, giving you the insight needed to target changes in areas of largest potential impact.
Ian Darbey is Support Supervisor in the Surgical Directorate in Beaumont Hospital and is currently completing an MSc in Data Analytics in National College of Ireland.